Video annotation is a foundational process in the realm of artificial intelligence, enabling AI models to recognize objects, actions, and dynamic scenarios within videos by labeling and describing video frames. Unlike static image annotation, video annotation captures the intricacies of motion and temporal changes, making it indispensable for applications that require an understanding of how scenes evolve, such as autonomous driving and surveillance systems. Precise video annotation data is pivotal for training high-performance AI vision systems, underpinning the entire development process of AI vision technologies.
This section will delve into the intricacies of video annotation, shedding light on how this intricate process forms the backbone of many AI-powered applications and services.
What is Video Annotation
Video annotation is the process of labeling video data to make it understandable and usable for machine learning algorithms. In practical terms, it involves assigning descriptive metadata to video frames, such as identifying objects, actions, events, or behaviors. This is achieved by marking various elements within each frame — for instance, drawing bounding boxes around objects, annotating the movements of people or vehicles, or labeling emotional expressions on faces. These annotations provide context and meaning to raw video footage, enabling AI systems to 'learn' from this data. This learning process is critical for developing accurate and efficient AI models that can perform tasks like object recognition, motion tracking, or behavior prediction. Essentially, through video annotation, we translate the complex, unstructured visual information of videos into a structured form that AI algorithms can understand and interpret, forming the foundation for various AI applications in real-world scenarios.
Video Annotation vs Image Annotation
Video annotation and image annotation are both pivotal in the realm of AI and machine learning, but they differ significantly in their application and complexity.
Image annotation involves labeling still images to help AI models recognize and interpret static visual data. This might include identifying objects, classifying scenes, or detecting boundaries and shapes within a single frame. Video annotation extends this concept to dynamic, moving sequences. It not only involves labeling objects or elements within each frame but also tracking their movement and changes over time. This temporal dimension adds a layer of complexity, as the AI must understand not just individual images but also the continuity and evolution of scenes and actions.
The benefits of video annotation are vast, particularly in its capacity to provide a more comprehensive and nuanced understanding of real-world scenarios. While image annotation gives AI a snapshot of the world, video annotation offers a full story, complete with progression and context. For example, in autonomous vehicle development, video annotation allows AI systems to understand not just the presence of pedestrians or other vehicles, but also their trajectory, speed, and likely next moves. This is crucial for predictive modeling and real-time decision-making. Similarly, in security and surveillance, video annotation helps in recognizing patterns of behavior over time, enabling systems to identify potential threats or anomalies more effectively than static image analysis could.
Furthermore, video annotation's ability to capture the nuances of human behavior and interactions opens up possibilities in fields like healthcare, where it can be used for patient monitoring and diagnostics, or in retail, for customer behavior analysis. The temporal data in videos allows for a deeper analysis of patterns and trends, providing insights that go beyond what static images can offer. In essence, while image annotation lays the groundwork for AI's visual understanding, video annotation builds upon it, offering a richer, more dynamic perspective that is vital for AI systems operating in a constantly changing and evolving world.
Video Annotation Techniques
Video annotation techniques can be broadly categorized into three types: manual, semi-automated, and automated, each with its unique characteristics, advantages, and limitations.
Manual Annotation
Manual annotation is the most traditional method, where human annotators label video data frame by frame. This approach is highly accurate since it relies on human judgment and attention to detail, which is particularly important for complex scenarios where contextual understanding is crucial. However, manual annotation is time-consuming and labor-intensive. It requires significant human resources and can be subject to human error, especially in long and tedious projects.
Semi-automated Annotation
Semi-automated annotation strikes a balance between manual labor and automation. In this approach, AI algorithms are employed to pre-annotate the video data, which is then reviewed and refined by human annotators. This method enhances efficiency, reducing the time and effort required compared to fully manual annotation. While it offers a faster turnaround and can maintain a high level of accuracy, the quality of semi-automated annotation heavily depends on the underlying AI's capabilities. There is also a need for continuous human oversight to correct and verify the AI-generated annotations, especially in complex or ambiguous situations.
Automated Annotation
Automated video annotation represents the cutting-edge technology in this field, where AI and machine learning algorithms are used to annotate video data entirely autonomously. This method boasts the highest efficiency, capable of processing large volumes of data at a speed unattainable by humans. However, the reliability and accuracy of automated annotation can vary, particularly in videos with complex scenes, varying lighting conditions, or intricate movements. While automated systems are continuously improving, they still require periodic training and validation by human experts to ensure their annotations are accurate and relevant.
Each of these techniques has its place in video annotation, depending on the project's requirements, the complexity of the task, and the desired balance between accuracy and efficiency. Manual annotation remains indispensable for projects where precision is paramount, semi-automated methods offer a practical compromise for moderately complex tasks, and automated annotation is ideal for large-scale projects where speed is essential, and the tasks are relatively straightforward.
Types of Video Annotation
Bounding Boxes
One of the most common forms of video annotation, bounding boxes involve drawing rectangular boxes around objects of interest in each frame. This technique is particularly useful for object detection and recognition tasks, such as identifying vehicles, people, or animals in a video. Bounding boxes are relatively simple to create and provide a good balance between accuracy and efficiency. However, their simplistic nature might not capture the exact shape or orientation of complex objects, which can be a limitation in certain applications.

3D Cuboids
Extending the concept of bounding boxes, 3D cuboids are used to annotate objects in a three-dimensional space. This method provides depth information, making it ideal for scenarios where understanding the spatial relationship of objects is crucial, such as in autonomous vehicle navigation or robotic manipulation tasks. While 3D cuboids offer a more detailed understanding of the environment, they require more time and expertise to annotate accurately compared to 2D bounding boxes.

Polygons
Polygon annotation involves drawing multi-sided shapes that closely conform to the contours of an object. This type of annotation is more precise than bounding boxes, particularly for irregularly shaped objects or when fine-grained object segmentation is needed. Polygons are widely used in scenarios where detailed object outlines are important, such as in agricultural monitoring for plant health assessment. The main drawback of polygon annotation is its time-consuming nature, requiring more detailed work by annotators.
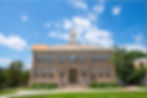
Skeletons
Skeleton annotation is used to map the human body or the structure of an object by annotating key points and connecting them with lines. This method is especially useful in motion analysis, posture detection, and activity recognition. Skeleton annotation helps in understanding the dynamics of movement, making it valuable in sports analytics, physical therapy, and human-computer interaction studies. However, accurately annotating skeletons can be challenging, particularly in videos with fast movements or where parts of the body are occluded.
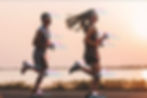
Lines and Splines
This type of annotation involves drawing lines or curves to represent linear features in a video, such as roads, boundaries, or trajectories. Lines and splines are useful in geospatial analysis, road safety studies, and tracking moving objects. They provide a simple yet effective way to represent direction and motion but might not capture the full complexity of certain scenarios, like crowded urban environments.
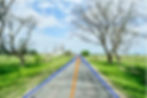
Semantic Segmentation
Semantic segmentation involves labeling each pixel in a video frame to a specific class or category, creating a pixel-wise map of different objects and elements. This detailed annotation is crucial for applications requiring a comprehensive understanding of the scene, such as autonomous driving, medical imaging, or landscape analysis. Semantic segmentation offers a high level of precision but is one of the most labor-intensive and time-consuming types of video annotation.
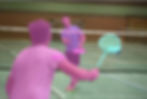
Enhance Your AI Model with BasicAI Video Annotation
BasicAI offers a comprehensive suite of video annotation services and tools, catering to a wide range of computer vision project needs. Our robust platform is equipped with versatile tools that support various forms of datasets, ensuring compatibility and flexibility regardless of the project's nature. This adaptability makes BasicAI an ideal choice for a variety of CV applications, from autonomous vehicles to surveillance systems. Beyond just providing tools, BasicAI excels in offering professional annotation services. We are recognized for delivering high-accuracy annotations, a crucial factor for the success of any AI model. What sets BasicAI apart is its cost-effective pricing structure, making our high-quality services accessible to a broad spectrum of clients, from startups to established enterprises. By combining advanced annotation tools with expert services, BasicAI stands as a one-stop solution for all video annotation requirements, ensuring that every project meets the highest standards of accuracy and efficiency.
Read Next
